New article on spatial autocorrelation and stochastic point processes
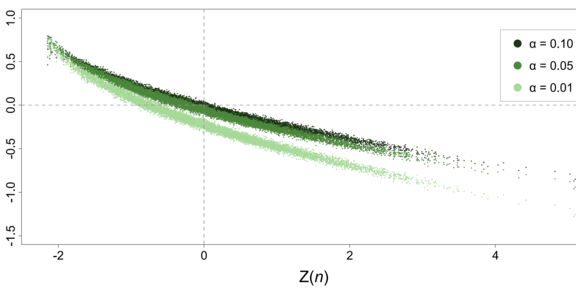
Estimating spatial autocorrelation is a statistical means of identifying and characterising structures in georeferenced data sets. Inference procedures for spatial autocorrelation statistics are based on the assumption of fixed configurations of spatial units. Sometimes, however, this assumption can prove disadvantageous, for example, when analysing social media posts or moving objects, where the geometric units to which attributes are assigned are also subject to a certain degree of randomness. In a newly published article in the journal Geographical Analysis by Jun.-Prof. Dr René Westerholt, it is investigated for the case of point geometries how the change from fixed to random spatial units affects inferences about global Moran's I, a popular spatial autocorrelation measure. Homogeneous and inhomogeneous Matérn and Thomas cluster processes are studied, and 10,000 random point patterns are simulated for each of these processes. These point processes are widely used to model a wide variety of phenomena such as the distribution of trees in forests, the study of crowds at large events and similar processes involving geometric randomness. Based on the simulations generated, three aspects are investigated that are central to inference about Moran's I: the null distributions of I under variation of the underlying geometries; the effect of the latter on critical values used to reject null hypotheses; and the effect of the presence of point processes on the statistical power of Moran's I. The results show that point processes affect all three properties. Inferences about spatial structure in relevant application contexts may therefore differ from conventional inferences when this additional source of randomness is taken into account.
Westerholt, R. (2022). A simulation study to explore inference about global Moran’s I with random spatial indexes. Geographical Analysis. DOI: 10.1111/gean.12349